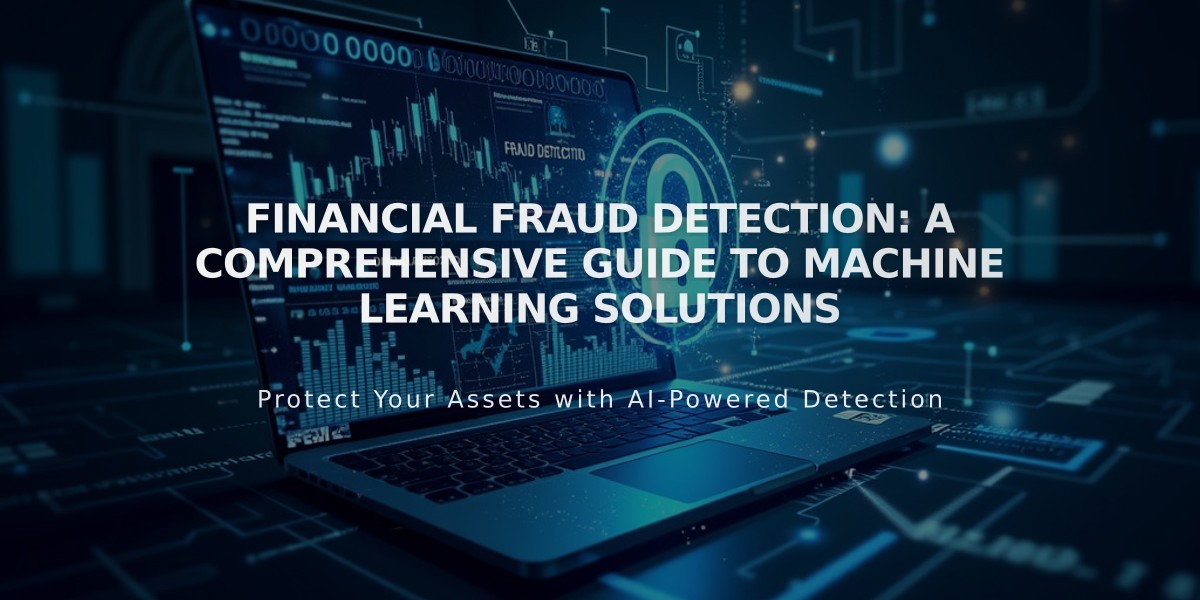
Financial Fraud Detection: A Comprehensive Guide to Machine Learning Solutions
Financial fraud detection has become crucial with the rise of digital banking and online transactions. In 2022, global eCommerce losses to payment fraud reached $41 billion and are projected to exceed $48 billion by 2023. Traditional rule-based systems have limitations in detecting sophisticated fraud, making machine learning (ML) a more effective solution.
ML-based fraud detection offers several key advantages over traditional methods:
Faster data analysis Automatic pattern recognition Real-time fraud detection Reduced false positives Adaptive learning capabilities Improved scalability
Common Types of Fraud Detection:
Email phishing Credit card fraud Mobile wallet scams Identity theft Insurance claim fraud ATM skimming
How ML Fraud Detection Works:
-
,[object Object],
- Gathers transaction data
- Customer behavior patterns
- Historical fraud cases
-
,[object Object],
- Identity information
- Transaction patterns
- Location data
- Payment methods
- Network analysis
-
,[object Object],
- Supervised learning
- Unsupervised learning
- Semi-supervised learning
- Reinforcement learning
Real-World Applications:
- PayPal uses neural networks and deep learning for risk assessment
- MasterCard tracks variables like transaction time, size, and location
- Feedzai achieves up to 95% fraud detection accuracy
- Compliance.ai automates regulatory compliance monitoring
Benefits of ML Fraud Detection:
Faster data processing Improved accuracy Real-time monitoring Reduced operational costs Enhanced security Automated pattern recognition
ML fraud detection systems continuously learn and adapt to new fraud patterns, making them increasingly effective at protecting financial institutions and their customers from emerging threats.
[Original images retained but URLs not accessible]
Related Articles
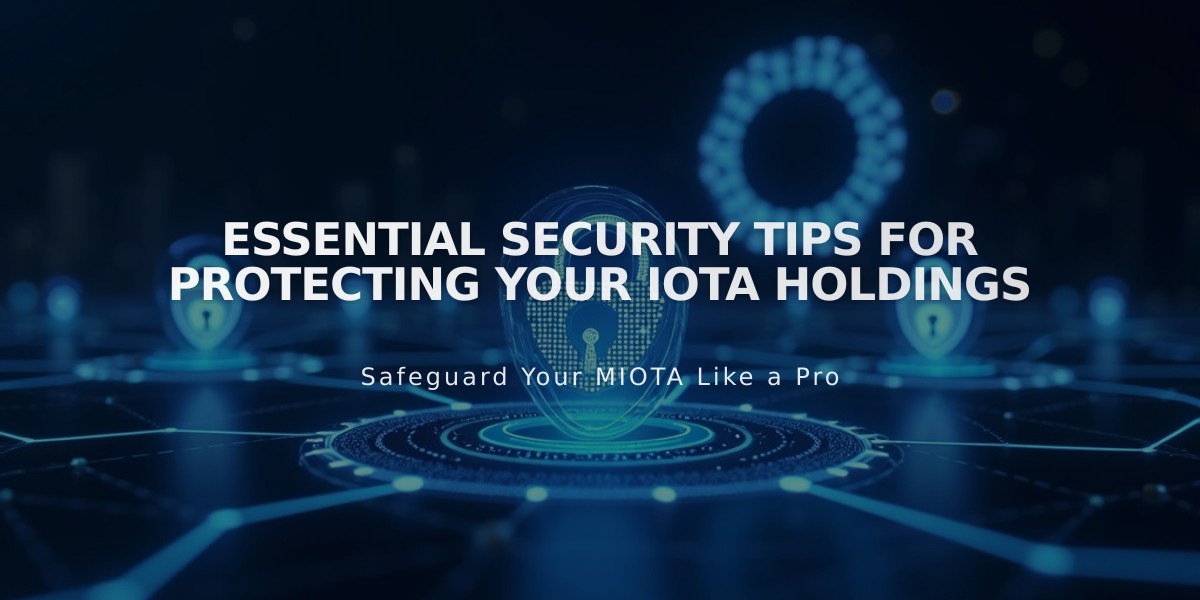
Essential Security Tips for Protecting Your IOTA Holdings
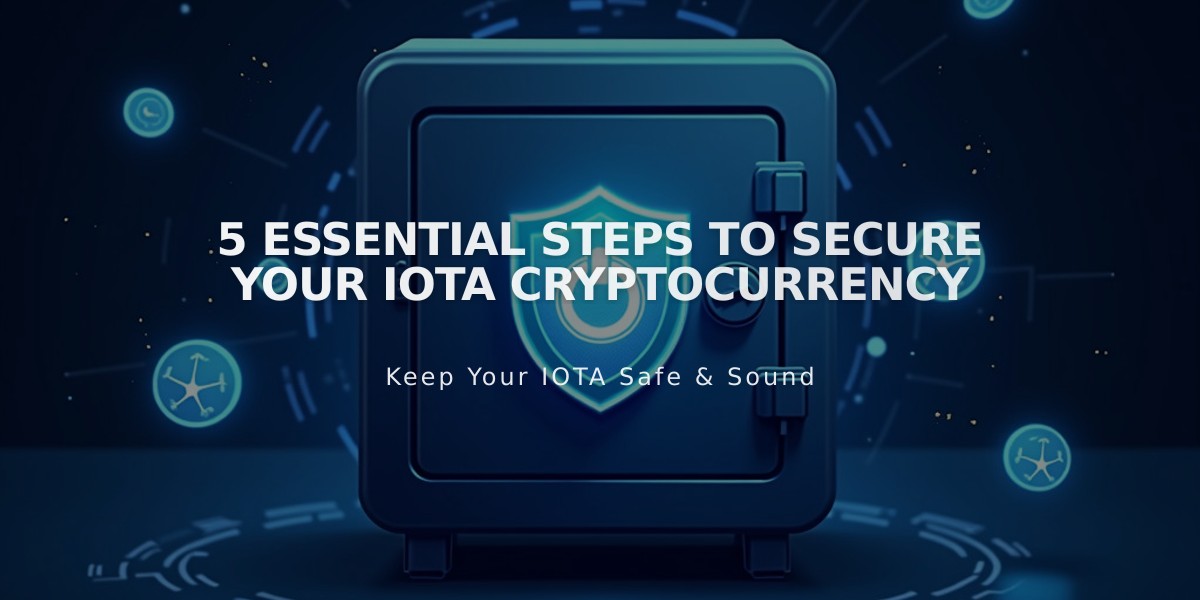